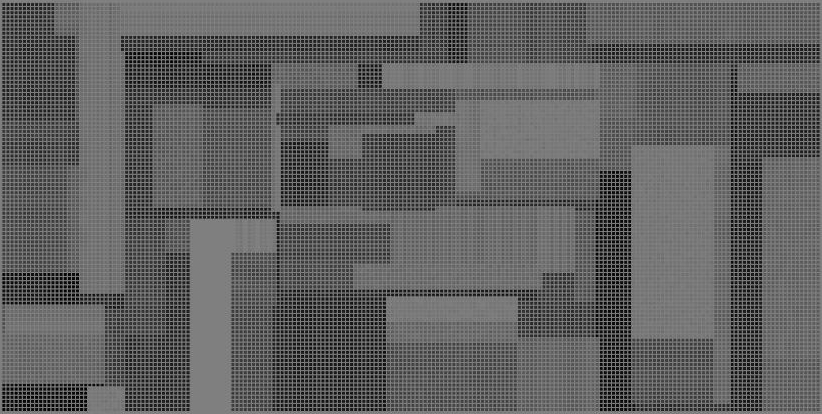
Creative constraints for data visualisation
Chris Martin - August 2022
One of the biggest challenges data visualisation designers face is the unbounded space of the possible. There are just so many possible ways any given dataset could be visualised.
When making data visualisations my head is constantly full of questions. Am I framing this in the ‘right’ way? Does this colour look right with the others? Have I picked the wrong chart type, maybe another would be better? Are these lines too wide or the points too big? And, so on …
Recently, I was thinking about how I face similar challenges when writing and producing music. The challenge of an unbounded space of possibilities. One of the strategies I have used to help me in a musical context is setting myself arbitrary creative constraints. For example, I might limit myself to using only one synthesiser on a track.
I started using constraints like this having been inspired by a chapter written by Dennis DeSantis in ’74 Creative Strategies for Electronic Music Producers’. A quote from Igor Stravinsky in the chapter really stuck with me. It beautifully encapsulates the essence of an idea that I think is very much transferable to data visualisation design.
“My freedom thus consists in my moving about within the narrow frame
that I have assigned to myself for each one of my undertakings. I
shall go even further: my freedom will be so much the greater and more
meaningful the more narrowly I limit my field of action and the more I
surround myself with obstacles. Whatever diminishes constraint
diminishes strength. The more constraints one imposes, the more one
frees oneself of the claims that shackle the spirit”.
Igor Stravinsky, Poetics of Music.
Experimenting with creative constraints in my own data visualisation practice
I started to wonder what using creative constraints in data visualisation would look like in practice. So, I decided to create myself a little experiment based on a couple of creative constraints:
- Pick a simple chart that you know well and remake it in ways you haven’t seen before.
- Don’t use any colour, just work in greyscale.
In the rest of this visual essay I will try to give you a sense of how my experiment went and what I learnt from it. I hope this will encourage you to consider using creative constraints in your own practice from time to time.
Here is the chart I chose to remake.
GDP per capita
2011 USD adjusted for inflation
Carbon emissions per capita
Tonnes of CO2 per year
As you read on, it will probably be helpful to remember three things about the chart.
- Each point in the scatterplot is a country and the size of the point is defined by the population of the country.
- On the x axis we see GDP per capita. This is used as an indicator of the quality of life of a ‘typical’ person in a given country. Based on the, at least partially, flawed assumption that the higher someone’s income the better their quality of life.
- On the y axis we see CO2 emissions per capita. This is of interest in its own right. It can also be used as an indicator of the overall environmental damage caused by the ‘typical’ person in a given country.
Looking beyond the data when thinking about aesthetics
I found that working without colour made it more challenging to create contrasts between the data and non-data elements of a chart. The non-data elements being things like the axis, the axis labels and ticks, and reference grid lines.
Normally, and without thinking about it, I would create these contrasts by using colour for data elements and greys for non-data elements. Working within my greyscale constraint meant I had to think harder than normal about how to de-emphasise the non-data elements in my chart. I wanted the non-data elements to sit comfortably in the background, while the data elements remained in the foreground as the focus of the reader’s attention.
I started thinking through in detail how much visual emphasis I wanted to give to each element of the chart. In the end, I thought of each element as having a visual emphasis rating between one and five (five being the maximum visual emphasis).
Visual Emphasis | Chart components |
---|---|
5. Maximum | Data points |
4. High | Annotations |
3. Medium | Axis lines and labels |
2. Low | Major gridlines and axis ticks |
1. Minimum | Minor gridlines and axis ticks |
As you can see in the table above, rather than giving all non data elements the same emphasis, I gave some a minimal or low emphasis. In practice, this meant that I used darker and lighter shades of grey, stroke width, and stroke length to create subtle contrasts between different non-data elements of the chart. Here is the result.
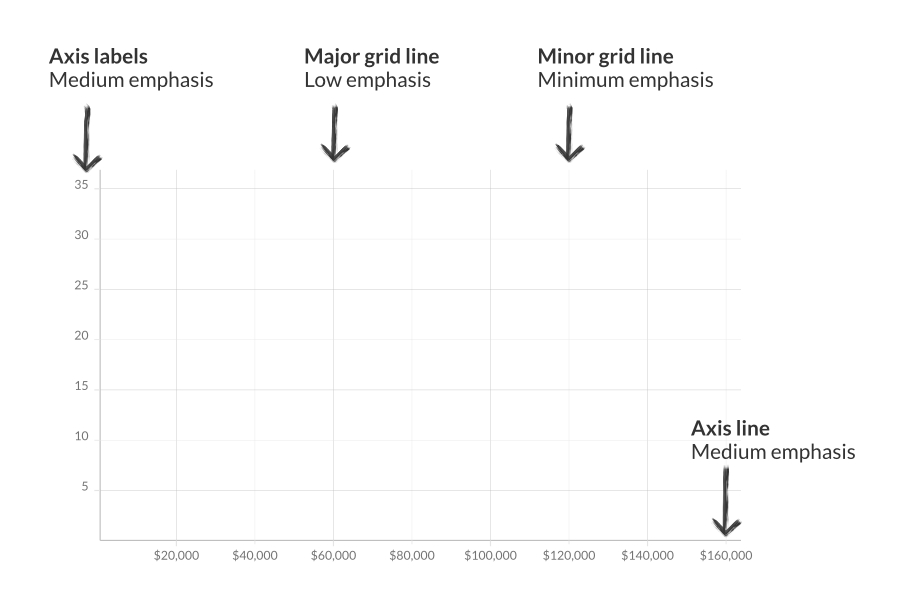
While it was time consuming to think through the aesthetics of the non-data elements in this level of detail, it did come with a longer-term pay off. I now have a consistent and reusable non-data background layer, which hopefully will give my charts a more distinct visual identity.
Using textures which connect to the topic of the chart
As I worked without colour, I also gravitated towards using textures to add visual interest to my charts, and to enhance the overall design aesthetic. I focused on adding textured backgrounds to annotations and tooltips.. Going through iterations of various chart versions enabled me to quickly experiment with different texture options. Discarding those options that didn’t work without much concern, as I hadn’t invested much time or energy in them.
Initially, I experimented a lot with different paper based textures. Here, I was just thinking about subtly enhancing the visual appearance of the chart. While making a version of the chart that highlighted the top 20 fossil fuel producing countries, I stumbled on an alternative approach.
I started thinking about how the textures could connect to the theme of the chart. This led me to creating a crude oil textured background for annotations. Oil is probably the fossil fuel people most commonly think of in the context of climate change. The dark liquid texture also worked well as a backdrop to the annotation text. The chart below shows this texture in the context.
GDP per capita
2011 USD adjusted for inflation
Carbon emissions per capita
Tonnes of CO2 per year
Over the last year or so, I have built a habit where I try to align the meanings and associations of colours with the message of a chart. Maybe it should have been obvious, but I had never considered doing something similar with texture. It was only working with my ‘no colour’ constraint that I made this connection. It is something I definitely want to carry though into my future work.
Thinking deeply about embedding context into a chart
When working normally, I would think about the different chart options that could be used with the data at hand. As well as how to use several potentially complementary charts together. Being constrained to work with just one chart (a scatter chart) made me think more deeply about how I embedded context into the chart. I couldn’t fall back on adding more charts to create a story with the data.
As I worked through various iterations of the chart, I realised a crucial piece of context was missing. What level of annual CO2 emissions per capita is compatible with keeping climate change impacts (in some sense) in check? Without this context the graph provides no sense of the scale of the decarbonisation challenge that we collectively face. So, I did some research and identified a recent and credible estimate of a sustainable level of annual carbon emissions per person (2.3 tonnes CO2e).
I went through several iterations of creating charts that integrated this additional context. These included adding a simple horizontal line, and visually representing countries above and below the 2.3 tonnes threshold in different ways.
It took me a bit of time to realise that I actually needed two versions of the chart to explain the importance of the 2.3 tonnes threshold. One which showed the countries where carbon emissions need to fall, and by how much. A second which shows the other side of the story, the countries where emissions could rise to a sustainable level as their economies grow. The two charts I settled on are shown below.
GDP per capita
2011 USD adjusted for inflation
Carbon emissions per capita
Tonnes of CO2 per year
GDP per capita
2011 USD adjusted for inflation
Carbon emissions per capita
Tonnes of CO2 per year
Reflecting on the process of creating these charts, I recognised that I often feel a pressure to find the one ‘best’ way to show the data. A single way that conveys a clear and unambiguous message. Working within the constraints freed me up from this pressure, and helped me to communicate a more nuanced message through two versions of the same chart. The primary difference between the two charts is just the axis extents, but this gives two very different views of the data.
Feeling free to use statistical techniques to enhance a chart
Working with only one chart also encouraged me to do some statistical modelling. I have some background in statistics, but I generally avoid using statistical modelling within my data visualisations. This is for two reasons: (1) I think I won’t have enough time to do ‘proper’ modelling; and, (2) I worry about how to communicate modelling in an accessible way.
Having exhausted other options within the creative constraints, I was left with the option of doing some statistics. This absence of alternatives helped me to overcome my mental barriers, and actually get on with doing some modelling with fewer concerns.
I started by doing some research about how in theory, a country's carbon emissions should change as its economy grows. The next step was to experiment with a few different models (using R). In essence, these models described the trajectory a country should theoretically take across the graph as its economy grows.
Then it was a case of thinking through how to visually explain the model, and its implications, in a way that is accessible for people without a statistical background. I’m not sure I got the whole way with this last step. Take a look at the chart below and see what you think. You can change between two views of the model and the data using the buttons above the chart.
GDP per capita
2011 USD adjusted for inflation
Carbon emissions per capita
Tonnes of CO2 per year
It felt like I learnt something really important from producing this last version of the chart. 'Your statistics and modelling do not need to be peer-reviewed academic research quality to enhance a data visualisation'. I definitely plan to put this on a post it note above my desk, so I don’t get stuck and completely avoid using stats and modelling.
Using creative constraints in your own data visualisation practice
I hope that reading about my experiences has piqued your interest in using creative constraints in your own data visualisation practice. The biggest things I got out of my experiment with creative constraints were:
- A way to focus my personal projects, which have tended to sprawl in the past!
- A great approach to use when learning new tools and technologies. In this case, working with the constraints helped me to focus on learning the basics of visualising data using Svelte and D3.
- A much needed reminder that you often have to work through the obvious ideas for visualising a dataset. Before you then get to the more innovative, potentially higher impact ideas.
- Some really helpful design ideas that I can reuse as I develop a consistent visual identity for my work.
There are so many different creative constraints you could set for yourself in your own experiments. As a starting point, here are just a few ideas for some different aspects of data visualisation practice.
Aspect of data visualisation practice | Ideas for creative constraints |
---|---|
Use of colour. | Use only colours found in a photo related to the topic of your chart. |
Use of shape. | Make a chart without any circles or open lines, only use squares and rectangles. |
Use of scale. | Make a chart which uses only logarithmic scales, and explain these to the reader. Find a dataset where it makes sense to do this. |
Non-data elements of the chart (axis, gridlines etc.). | Remake a version of one of your previous charts, but without an axis. |
Choice of chart. | Show the same dataset using as many different chart types as possible. Make sure to include chart types you don’t think will work. |
Analysis and statistics. | Apply an analytical technique you haven’t used before, and integrate the findings into a chart. Remember you don’t need to master the technique to use it in your experiment. |
Technologies. | Only use one tool (e.g. Datawrapper, Figma …) to create a chart. Or, maybe just use a pen and paper to hand-draw a chart. |
Do get in touch if reading about my experience of using creative constraints sparked off any ideas for you. Or, if you try any of the ideas above. It would be great to hear from you, and see where you get to with your own experiments.
Acknowledgements
- The pattern at the top of the page was produced with code adapted from Art from Code by Danielle Navarro.
- The photograph of oil used in the annotation background texture was taken by Tom Barrett.
- Feedback and ideas throughout the development and writing process from the Elevate Data Viz community.